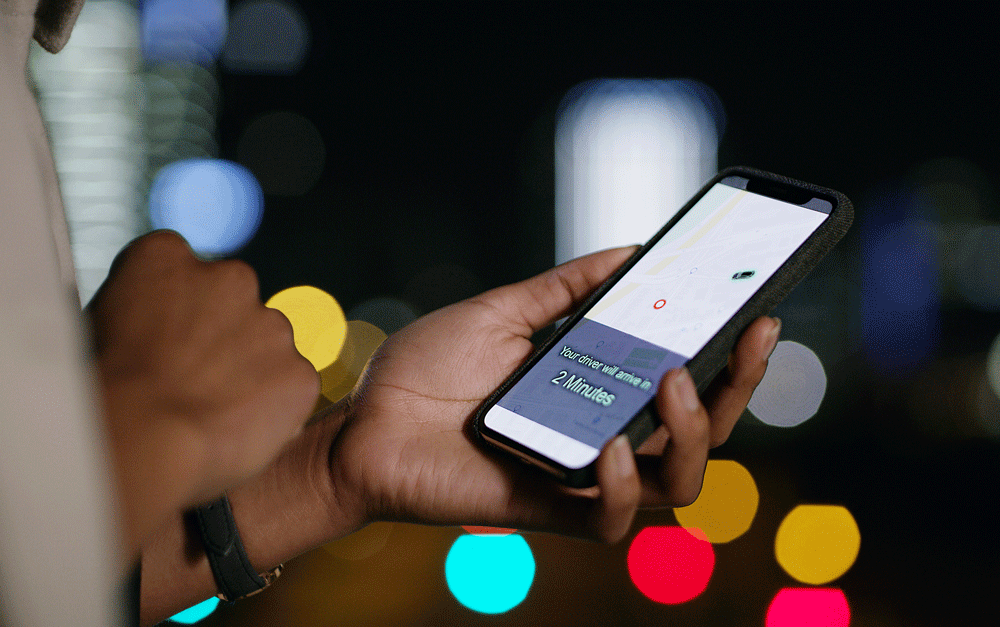
Doctoral student Xiaojian Zhang is working to reduce social inequalities by analyzing biases in mobile device location data gathered and tracked from technologies such as mobile phones or other GPS-enabled devices.
Mobile device location data has become an important tool for transportation researchers and professionals working to improve human mobility analysis. For example, transportation professionals use mobile device location data to understand traffic patterns, including how people move from one location to another. This type of data can help transportation professionals forecast travel and run simulations that help them create strategies to improve various aspects of transportation. Human mobility analysis refers to the investigation of data sets showing how mobile or smartphone users, for example, interact within their geographical settings.
However, this type of data faces some challenges, especially when it relates to disadvantaged populations and communities. The reason? Because they are usually underrepresented in mobile location data. If this data is used in policymaking, then funding that is allocated for transportation may unintentionally get skewed towards advantaged populations or communities, worsening the inequalities already in existence.
Zhang and the coauthors, Dr. Xilei Zhao and Dr. Xiang ‘Jacob’ Yan of the UF Department of Civil and Coastal Engineering, proposed a methodology to methodically explore how these biases show up in the data or results. Specifically, the methodology considers raw data preprocessing, home location inference, and bias analysis. It also focuses on identifying two potential biases: sociodemographic and geographic. The researchers tested the methodology on real-world datasets gathered from the entire state of Florida.
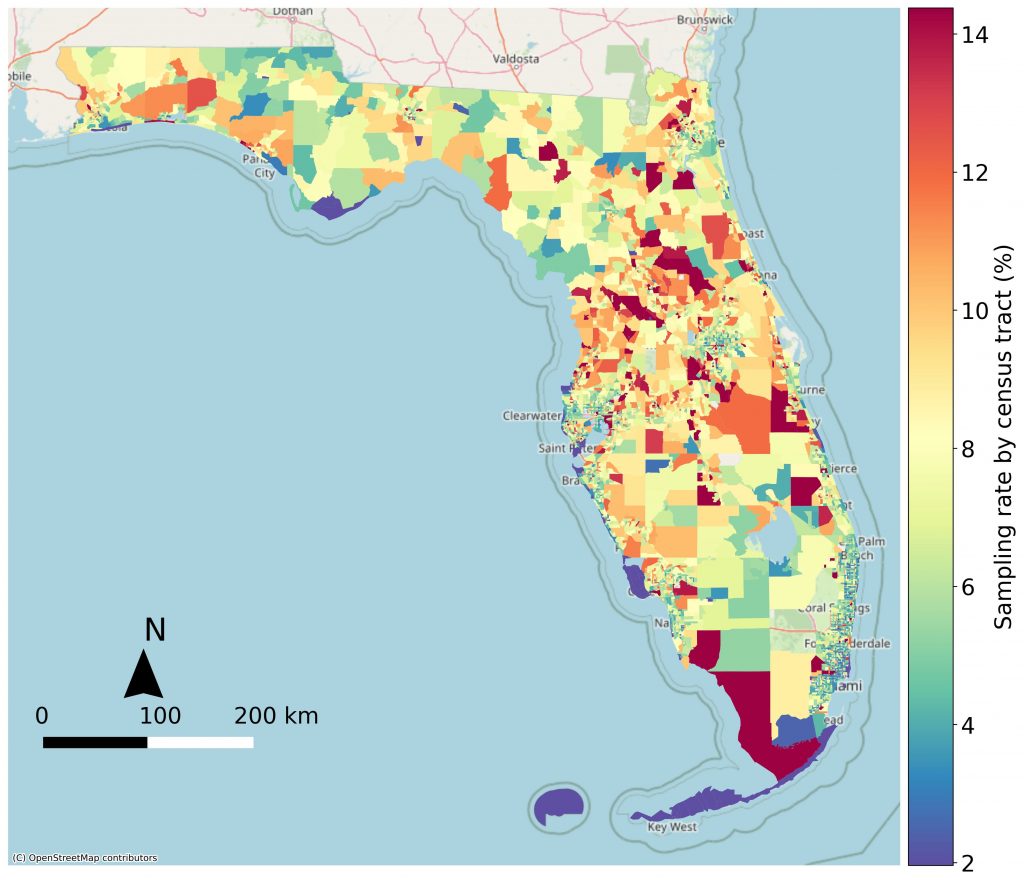
The results revealed that the number of mobile devices sampled correlated with the size of the population as per the most recent U.S. Census information. The study also showed that the groups most likely to be undersampled were those living in areas that were mostly populated by lower-income, non-white, and Hispanic populations who were also older.
The information gleaned from this study can help transportation engineers and planners create policies that are not biased toward advantaged communities, thus creating a more equitable approach that addresses the needs of all populations.
Zhang presented the results of his findings at the 103rd Annual Meeting of the Transportation Research Board.