
Micro-mobility such as e-scooters and dockless bicycles have gained popularity in recent years and are widely seen in the urban transportation system. Because most of these lightweight devices are used in and around downtown areas, they have the potential to not only complement public transit, but also to relieve traffic congestion by replacing automobiles that can often cause congestion in a city’s core.
But with limited research on modeling and analyzing the impacts of micromobility on the existing transportation system, including quantifying its impact on mitigating congestion, there are still questions to be answered. For example, what market penetration rate of e-scooters can achieve an effective reduction in congestion? What are the impacts of availability of parking on e-scooter usage? Which roads or streets need connected bike lanes in order to ensure the safety of e-scooters and promote the modal shift from car to e-scooter?
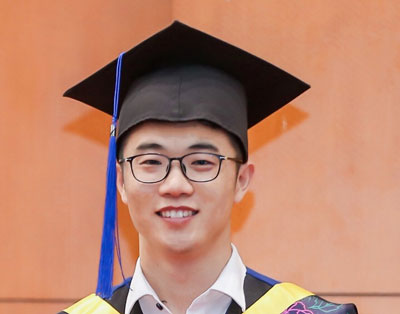
Yiming Xu, a doctoral student at the UFTI, is working on answering these and other questions. He will be using publicly available data and machine learning in a STRIDE-funded project titled “Micromobility as a Solution to Reduce Urban Traffic Congestion”.
“My role includes raw data collection such as scraping the micromobility usage data, collecting the socio-demographic and land-use data, and data processing such as extracting micromobility trips from the massive and messy raw data,” he said. “I will also be developing machine learning models and interpreting the models to explore the relationships between e-scooter travel demand and other important features, including traffic conditions, time of day, availability of bike lanes.”
Xu is working on this project under the guidance of his doctoral adviser, Dr. Xilei Zhao, assistant professor in the UF Department of Civil & Coastal Engineering. Dr. Zhao is also the principal investigator on the project.
Currently, the research team has found that e-scooter travel demand is high in downtown areas where congestion often occurs. And built environment variables such as riding environment quality, parking density, and hotel density have positive effects on e-scooter travel demands.

It is expected that the project will produce a historical e-scooter database to facilitate micromobility research, develop new machine-learning methodologies to model and interpret travel demand, and improve traffic simulation models to account for this new travel mode with different scenarios. The research team will also develop an interactive decision-support tool for local stakeholders to help with decision-making related to micromobility. Additionally, research results will help guide a set of policy interventions for promoting the shift away from automobiles to lightweight devices such as e-scooters or dockless bicycles.